top of page

Seeing Is Believing: Fake News in Social Media
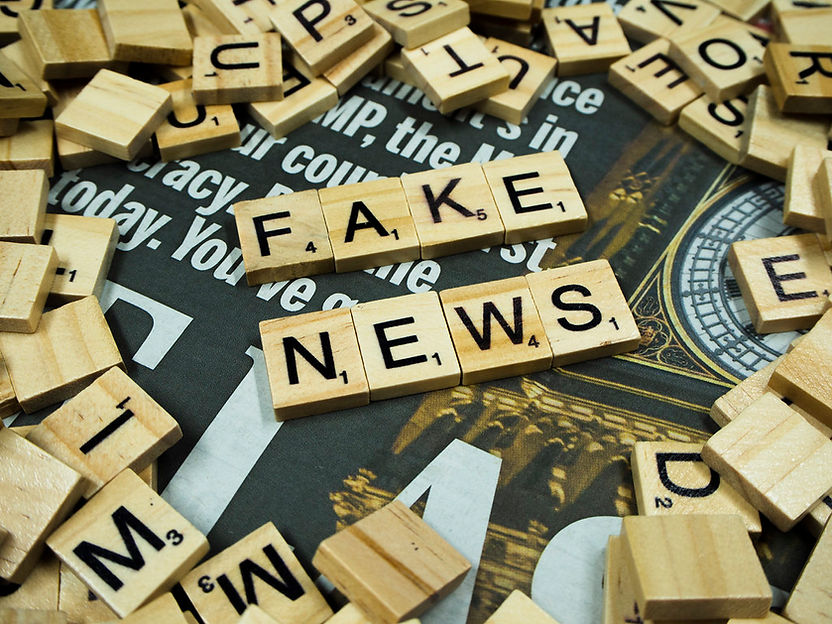
There is an old saying that “a lie can travel halfway around the world while the truth is still putting on its shoes” (Chokshi, 2017, para. 1). Thanks to social media, misinformation can spread at lightning speed. Since the 2016 presidential campaign, the term “fake news” has taken on new cultural and political significance. Bovet and Makse (2019) defined fake news as “fabricated information that disseminates deceptive content, or grossly distorts actual news reports, shared on social media platforms” (p. 2). Ahead of the election, “super-sharers” on social media, who were disproportionately conservative, accounted for 80% of fake news sharing or exposure (Grinberg, Joseph, Friedland, Swire-Thompson, & Lazer, 2019). Numerous fabricated reports were shared, including that Pope Francis had endorsed Donald Trump for president or that Hillary Clinton had sold weapons to ISIS (Ritchie, 2016). In the aftermath of President Trump’s victory, U.S. intelligence agencies concluded with a high degree of confidence that Russian operatives had exploited social media to interfere with the presidential campaign (Jamieson, 2018). As a result, some members of the public have wondered whether fake news helped elect the president and to what extent it may have swayed some voters away from Clinton. Researchers have conducted several studies on the phenomenon. In this literature review, I will analyze research on fake news to link several common threads: the tendency of users to favor information that aligns with their belief system, the role of Facebook’s algorithms in spreading falsehoods, the rise of hyperpartisan websites, and the advantage fake news gave to Trump. Finally, I will explore a dangerous new front in the fight against online falsehoods.
Communication Context and Rationale
Before looking into how fake news spreads online, it is important to note why an examination of this issue is critical. The full extent of Russian interference—or, as Jamieson (2018) called it, Russia’s “cyberwar”—remains challenging to determine with precision. However, what is certain is there is a receptive audience for fake news online. According to Gottfried and Shearer (2016), 62% of adults in the United States get their news from social media. Even if a few thousand people in key swing states like Wisconsin or Michigan had been influenced by fake news before voting, it could have been enough to sway the 2016 presidential election. In Michigan, Trump’s margin over Clinton was 10,704 votes (Jamieson, 2018). Prior to the 2020 presidential election, it is crucial for voters to be aware that what they see online may not be true and may instead be an attempt to manipulate them to vote or think in a certain way.
Research Methods and Key Findings
After reviewing the literature on the issue, several common research methods and communication theories emerged. Prior research studies have involved conducting message system analysis, or content analysis, of Facebook posts and tweets that researchers deemed fake or deliberately misleading. Allcott and Gentzkow (2017) conducted the first-known study on the impact of fake news in 2016, drawing on a database of 156 election-themed news stories that they concluded were fabricated. Allcott and Gentzkow also conducted a 1,200-person survey following the election to try to determine whether voters recalled some of the fake stories that users were spreading online. They concluded that because pro-Trump fake news had largely been seen by people who were already inclined to vote for him, they would expect the falsehoods to have a small impact on voting decisions. In another study, Bovet and Makse (2019) examined 30 million tweets that contained a link to a news story in the 5 months before the election. Based on an independent classification of the linked sites, they determined that 25% of the tweets contained fake or extremely biased news. Guess, Nagler, and Tucker (2019) linked a survey of 3,500 people in a national sample in the United States to the respondents’ history on Facebook to determine how much fake news they might have shared. Perhaps reassuringly, they concluded that the vast majority of users on that platform did not share fake news at all in the lead-up to the 2016 election. Guess, Nyhan, and Reifler (2018) surveyed 2,525 Americans who consented to provide their web traffic data from their computers, and they estimated that one in four Americans visited a fake news site in the 2 months before the election day.
Communication Theories
Theory can help provide answers about why people share fake news. Festinger (1957) defined cognitive dissonance as the psychological state in which our beliefs contradict our attitudes or actions. To restore balance, we need our beliefs and attitudes to be consistent. One way to achieve that is through confirmation bias, the “tendency to give more weight to information that confirms one of our preexisting beliefs” (McIntyre, 2018, p. 173). Bovet and Makse (2019) attributed the sharing of fake news to confirmation bias. Users are more likely to share, like, or comment on stories that affirm their beliefs. Ashley, Roberts, and Maksl (2018) affirmed the role of confirmation bias in allowing falsehoods to flourish; they noted that users “may have shared the stories without reading them or failed to fact-check them because of this tendency to believe information with which they agreed. They wanted the stories to be true and so behaved as if they were” (p. 152). Another study called this action congruency, concluding that political affinity drove the sharing of fake news sources (Grinberg et al., 2019).
Ashley et al. (2018) also mentioned two-step flow theory, which suggests that information, particularly political ideas, flows from mass media to community opinion leaders and on to members of the public. Because social media sites are set up to allow users to share or retweet material from the sources they follow, fake news can spread quickly.
Jamieson (2018) agreed with the role two-step theory played in the 2016 election, but she also noted another theory that she believed bolstered her claim that the Kremlin helped elect Donald Trump. In particular, she stressed the role of agenda-setting theory, which suggested that the media help shape the political narrative (McCombs & Shaw, 1972). Jamieson argued that because Russian trolls succeeded in getting pro-Trump themes to trend on Twitter, journalists would consider those trending topics more newsworthy and set the news agenda accordingly. For example, less than an hour after the infamous Access Hollywood tape of Trump having a lewd conversation about women emerged, WikiLeaks released emails stolen from Clinton Campaign Manager John Podesta. Journalists began covering these revelations with equal weight to the Access Hollywood tape to appear politically objective. Jamieson argued this effort at equal time created a false equivalence between the stolen emails and Trump bragging about sexual assault.
Another component of agenda-setting involves framing, in which the media focuses on certain angles and “tells audiences how to think about issues” (as cited in Jamieson, 2018, p. 44). Russian trolls had worked overtime to paint Clinton as disingenuous and corrupt. By making the WikiLeaks content more salient, the media helped underscore the belief that Clinton had something to hide, furthering perceptions that she was scandal-plagued and dishonest. This decision gave another political advantage to Trump. Benkler, Faris, and Roberts (2018) also noted how right-wing outlets such as Breitbart and InfoWars framed the issue of immigration in terms of Islamophobia and threats to national security, mirroring Trump’s talking points.
Facebook’s Role
The Frontline episode “The Facebook Dilemma” blamed the proliferation of fake news on the company’s proprietary algorithm, which ranks and displays content on a user’s news feed. These under-the-hood calculations push “engaging content” to the top of users’ home pages (Jacoby, Bourg, & Priest, 2018), and stories may go higher up the feeds if they have more likes, comments, or shares. Because people tend to like posts that align with their worldview or affirm their confirmation bias, Facebook has effectively created filter bubbles in which some people are only ever exposed to one political orientation (Solon, 2016). For example, if liberal-leaning voters only see posts that attack Trump, this echo chamber isolates them from other points of view and amplifies the negative posts they share, some of which may be untrue. Benkler et al. (2018) agreed that Facebook’s algorithm rewards what they called “hyperpartisan bullshit” and false content (p. 10). Facebook is also accused of acting as an “amplification service for websites that otherwise receive little attention online, allowing them to spread propaganda during the 2016 election” (Dreyfuss & Lapowsky, 2019, para. 6). Because of the social network’s reach, little-known sites have found a platform to spread their content to the masses.
Following intense public backlash against the company, Facebook announced on April 10, 2019, that it would start punishing groups that share misinformation by preventing them from showing up in large numbers of users’ news feeds (Dreyfuss & Lapowsky, 2019). Many of these groups are blamed for spreading fake news during the 2016 election. It also announced a partnership with The Associated Press to begin fact-checking online videos, saying it will label content with “Trust Indicators” to give users context about the veracity of reporting (Dreyfuss & Lapowsky, 2019).
Post-Truth
For some people, however, the truth may not matter. McIntyre (2018) explained that we live in a post-truth era, where “objective facts are less influential in shaping public opinion than appeals to emotion and personal belief” (p. 5). He argued that information could be introduced within a political framework to support one meaning of truth over another. For example, shortly after Trump’s inauguration, Senior Adviser Kellyanne Conway said that White House Press Secretary Sean Spicer had given “alternative facts” regarding the size of the crowd (McIntyre, 2018, p. 6). Much like confirmation bias, this newly coined phrase suggested that other information can be used to challenge certain facts that may be hostile to one’s preferred point of view. Benkler et al. (2018) affirmed this notion of post-truth, suggesting that audiences and Internet users have a much more difficult time today differentiating fact from fiction: “They are left with nothing but to choose statements that are ideologically congenial or mark them as members of the tribe” (p. 37). The authors added that, in the absence of truth, the most entertaining or shocking conspiracy theory often fills the void.
Right-Wing Media
One place that conspiracy theories thrive is in far-right online outlets. For years, conservatives have branded the mainstream media as the “liberal media.” Starting in the 1990s, many Republicans began speaking openly about how the media were on the side of the Clintons (McIntyre, 2018). Conservative talk radio and the rise of Fox News gave Republicans an outlet to echo their frustrations and give voice to their beliefs. Benkler et al. (2018) divided the mass media into two distinct camps: “the right and the rest” (p. 225). They argued that the left has no real equivalent to far-right sites such as Breitbart, InfoWars, Truthfeed, and Gateway Pundit, which traffic in misleading half-truths or made-up stories. They conducted a content analysis on two million articles written during the 2016 election, including fake and real news stories, and concluded that they were unable to find an example of a fake news story that started on the left and took hold for any period.
Of course, left-wing advocacy sites do exist: Daily Kos, Talking Points Memo, and Media Matters are a few examples. However, these sites tend to be more of an echo chamber for liberal and progressive themes than outlets for fake news (Benkler et al., 2018). Meanwhile, InfoWars promoted a conspiracy theory that the Sandy Hook shooting was a hoax involving child actors (Hemmer, 2018). Even Fox News pushed a debunked conspiracy claiming that Democratic National Committee staffer Seth Rich was killed after he allegedly leaked emails to WikiLeaks. Fox News host Sean Hannity embraced this story about Rich on his nightly show, which attracts millions of viewers. He also routinely promotes the conspiracy of a “deep state” in which government officials are actively trying to undermine Trump’s presidency (Hemmer, 2018). President Trump is no stranger to promulgating these conspiracy theories. Among other things, he launched the “birther” movement, which claimed that President Obama was not born in the United States, and he implied that Ted Cruz’s father was involved with the Kennedy assassination (Benkler et al., 2018).
Pro-Trump Fake News
Although no study has definitively proven that Trump won the election because of Russian meddling, all the studies I reviewed concluded that he was the primary beneficiary of fake news. Allcott and Gentzkow (2017) determined that “fake news was both widely shared and heavily tilted in favor of Donald Trump” (p. 212). They found 115 pro-Trump fake stories that were shared on Facebook 30 million times, compared to 41 pro-Clinton false stories that were shared 7.6 million times. Guess et al. (2018) similarly found that people saw an average of 5.45 articles from fake news websites before the election, nearly all of which were skewed toward Trump. Guess et al. (2019) noted that the Republicans in their sample shared more fake news stories than the Democrats, and the sources were mostly positive for Trump.
Surprisingly, one reason fake news primarily benefited Trump had little to do with its authors wanting to see him elected. Instead, these stories served as “clickbait” after the authors noticed that pro-Trump stories, or stories that attacked Clinton, received the most clicks. McIntyre (2018), Allcott and Gentzkow (2017), and Ashley et al. (2018) all concurred that one of the primary motivations for the creation of fake news was financial gain for the creators. Fictitious stories about Trump generated more engagement online, resulting in increased revenue through Google AdSense and Facebook’s advertising platform. Higgins, McIntire, and Dance (2006) interviewed a student from the country of Georgia who created fake news stories on social media. He said posts about Clinton did not generate much interest, but fake reports that were skewed toward Trump did.
While noting that Trump was the primary beneficiary of fake news, researchers are somewhat conflicted about the actual impact on election results. Allcott and Gentzkow (2017) argued that fake news is unlikely to have changed the outcome of the 2016 presidential election. Benkler et al. (2018) similarly found no evidence that Russian trolls or fake news helped sway the vote. However, Jamieson (2018) suggested the opposite: She argued that by targeting content to align with Trump’s electoral interests, Russian trolls likely helped to elect him as president. She justified her findings “based on the preponderance of evidence” (p. 14). Regardless, Ashley et al. (2018) wrote that it is difficult to determine with certainty how many people saw fake news and to what extent, if any, it influenced voting decisions.
The Mueller Report
As the public and the mass media scrutinize the redacted release of special counsel Robert Mueller’s report on Russian interference, there has emerged new information about the extent of Moscow’s involvement in trying to sway the vote. Mueller (2019) concluded, “The Russian government interfered in the 2016 presidential election in sweeping and systematic fashion” (p. 9). The report detailed Russian efforts to hack voting technology in the United States, to target election administrators in several states, and to infiltrate the computers of people linked to the Democratic campaign. In doing so, the report says Russia “stole hundreds of thousands of documents from the compromised email accounts and networks” (Mueller, 2019, p. 13). The report was not the first time Mueller’s team had pointed fingers at Russia. In 2018, the special counsel indicted 12 Russian officers for election interference, and he provided evidence of the role that troll factories and paid agents played in shaping the political narrative online (Benkler, 2018). One individual who loomed large in that report is WikiLeaks founder Julian Assange. In his report, Mueller (2019) noted Assange’s statements about the murdered DNC staffer Seth Rich “implied falsely that he had been the source of the stolen DNC emails” (p. 48). On April 11, 2019, British police arrested Assange in London after the government of Ecuador withdrew his asylum. He awaits extradition to the United States on charges of attempting to hack into classified material on U.S. government computers in 2010 (Savage, Goldman, & Sullivan, 2019). Assange may face additional charges; the indictment does not mention WikiLeaks’ more recent publishing of Democrats’ emails, which authorities have said Russia stole to help influence the 2016 presidential election (Savage et al., 2019).
Deepfakes and Artificial Intelligence
As technology has advanced, a new front in the fight against fake news has emerged. Machine learning algorithms and artificial intelligence (AI) have given rise to so-called “deepfakes.” Chesney and Citron (2019) described these as “highly realistic and difficult-to-detect digital manipulations of audio or video [in which] it is becoming easier than ever to portray someone saying or doing something he or she never said or did” (para. 1). For example, using a laptop computer, a person can manipulate a sound bite from President Trump saying he did collude with the Russians. With the aid of AI software, the face of one subject can be morphed on top of another to create realistic results (Ellis, 2018). Celebrities, world leaders—anyone, for that matter—can be smeared, seemingly by their own words. Because celebrities and politicians have so much visual information available online, they could become easy targets (Ellis, 2018). In his book, Messing with the Enemy: Surviving in a Social Media World of Hackers, Terrorists, Russians, and Fake News, Watts (2018) also discussed this emerging threat: “Data dominance will enable machine learning to create highly convincing hoaxes, propelling video smears and audio pronouncements” (p. 233). He also declared, “Machine-learning advancements can be nuclear weapons for information warfare” (p. 232). Chesney and Citron (2019) added, “As deepfake technology develops and spreads, the current disinformation wars may soon look like the propaganda equivalent of the era of swords and shields” (para. 1). All of this adds a worrisome, and potentially dangerous, dimension to the fight against fake news.
Although AI may make combatting falsehoods online even more complicated, technology may help play a hand in limiting their proliferation. Facebook and Twitter have vowed to do a better job of stopping fake news on their platforms. Facebook points to promising AI tools that can help detect fake news (Waugh, 2019). These tools refer posts to humans who fact-check them. Twitter also says it is investing in AI technology to try to fight the problem.
Conclusion
Jamieson (2018) concluded her book with a dire warning: the United States is vulnerable, and what happened in 2016 could happen again. For years, social media sites took a hands-off approach and did not employ fact checkers, allowing untruths to flourish (Solon, 2016). Recently, Facebook and Twitter have been stepping up efforts to suspend suspicious accounts that spread falsehoods; however, given the public’s tendency to like and share stories that align with their beliefs, there will continue to be a market for fake news. The rise of hyperpartisan news sites will not make the problem go away anytime soon, and that poses a threat to the pursuit of truth. For these reasons, it is vital to continue researching the phenomenon of fake news on social media. As George Orwell ominously noted, “The very concept of objective truth is fading out of the world. Lies will pass into history” (as cited in McIntyre, 2018, para. 1).
References
Allcott, H., & Gentzkow, M. (2017). Social media and fake news in the 2016 election. Journal of Economic Perspectives, 31(2), 211–236. doi:10.1257/jep.31.2.211
Ashley, S., Roberts, J., & Maksl, A. (2018). American journalism and fake news. Santa Barbara, CA: ABC-CLIO.
Benkler, Y., Faris, R., & Roberts, H. (2018). Network propaganda: Manipulation, disinformation, and radicalization in American politics. New York, NY: Oxford University Press.
Bovet, A., & Makse, H. A. (2019). Influence of fake news in Twitter during the 2016 US presidential election. Nature Communications, 10(1), 1–14. doi:10.1038/s41467-018-07761-2
Chesney, R., & Citron, D. (2019). Deepfakes and the new disinformation war: The coming age of post-truth geopolitics. Foreign Affairs, 98(1), 147–155. Retrieved from http://proxy.foley.gonzaga.edu/login?url=https://search-proquest-com.proxy.foley.gonzaga.edu/docview/2161593888?accountid=1557
Chokshi, N. (2017, April 26). That wasn’t Mark Twain: How a misquotation is born. The New York Times. Retrieved from https://www.nytimes.com/2017/04/26/books/famous-misquotations.html
Dreyfuss, E., & Lapowsky, I. (2019, April 10). Facebook is changing news feed (again) to stop fake news. Wired. Retrieved from https://www.wired.com/story/facebook-click-gap-news-feed-changes/
Ellis, M. (2018, November 21). Deepfakes explained: The AI that’s making fake videos too convincing. Retrieved from https://www.makeuseof.com/tag/what-are-deepfakes-explained/
Festinger, L. (1957). A theory of cognitive dissonance. Stanford, CA: Stanford University Press.
Gottfried, J., & Shearer, E. (2016). News use across social media platforms 2016. Retrieved from http://www.journalism.org/2016/05/26/news-use-across-social-media-platforms-2016.
Grinberg, N., Joseph, K., Friedland, L., Swire-Thompson, B., & Lazer, D. (2019). Fake news on Twitter during the 2016 U.S. presidential election. Science, 363(6425), 374–378. doi:10.1126/science.aau2706
Guess, A., Nagler, J., & Tucker, J. (2019). Less than you think: Prevalence and predictors of fake news dissemination on Facebook. Science Advances, 5(1) 1–8. doi:10.1126/sciadv.aau4586
Guess, A., Nyhan, B., & Reifler, J. (2018). Selective exposure to misinformation: Evidence from the consumption of fake news during the 2016 U.S. presidential campaign. Retrieved from https://www.dartmouth.edu/~nyhan/fake-news-2016.pdf
Hemmer, N. (2018, January 12). How Breitbart became just another right-wing Trump cheerleader. The Washington Post. Retrieved from https://www.washingtonpost.com/outlook/how-breitbart-became-just-another-right-wing-trump-cheerleader/2018/01/12/fa90bec0-f6f6-11e7-a9e3-ab18ce41436a_story.html?utm_term=.6ca71e60852a
Higgins, A., McIntire, M., & Dance, G. (2016, November 25). Inside a fake news sausage factory: “This is all about income.” The New York Times. Retrieved from https://www.nytimes.com/2016/11/25/world/europe/fake-news-donald-trump-hillary-clinton-georgia.html
Jacoby, J., Bourg, A., & Priest, D. (Producers). (2018, December 11). The Facebook dilemma [Television series episode]. In Frontline. Washington, DC: Corporation for Public Broadcasting. Retrieved from https://www.pbs.org/wgbh/frontline/film/facebook-dilemma/
Jamieson, K. (2018). Cyberwar: How Russian hackers and trolls helped elect a president: What we don’t, can’t, and do know. New York, NY: Oxford University Press.
McCombs, M., & Shaw, D. (1972). The agenda-setting function of mass media. Public Opinion Quarterly, 36(2), 176–187. doi: 10.1086/26799
McIntyre, L. (2018). Post-truth. Cambridge, MA: The MIT Press.
Mueller, R. (2019). Report on the investigation into Russian interference in the 2016 presidential election. Washington, DC: U.S. Department of Justice.
Neuman, W. L. (2014). Social research methods: Qualitative & quantitative approaches (7th ed.). Tamil Nadu, India: Pearson Education Limited.
Ritchie, H. (2016, December 30). Read all about it: The biggest fake news stories of 2016. CNBC. Retrieved from https://www.cnbc.com/2016/12/30/read-all-about-it-the-biggest-fake-news-stories-of-2016.html
Savage, C., Goldman, A., & Sullivan, E. (2019, April 11). Julian Assange arrested in London as U.S. unseals hacking conspiracy indictment. The New York Times. Retrieved from https://www.nytimes.com/2019/04/11/world/europe/julian-assange-wikileaks-ecuador-embassy.html
Shakespeare, W. (n.d.). The Tempest. Retrieved from https://www.folgerdigitaltexts.org/download/pdf/Tmp.pdf
Solon, O. (2016, November 10). Facebook’s failure: Did fake news and polarized politics get Trump elected? The Guardian. Retrieved from https://www.theguardian.com/technology/2016/nov/10/facebook-fake-news-election-conspiracy-theories
Watts, C. (2018). Messing with the enemy: Surviving in a social media world of hackers, terrorists, Russians, and fake news. New York, NY: HarperCollins.
Waugh, R. (2019, March 6). How artificial intelligence has the ability to detect fake news. The Telegraph. Retrieved from https://www.telegraph.co.uk/technology/information-age/can-artificial-intelligence-detect-fake-news/
bottom of page